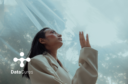
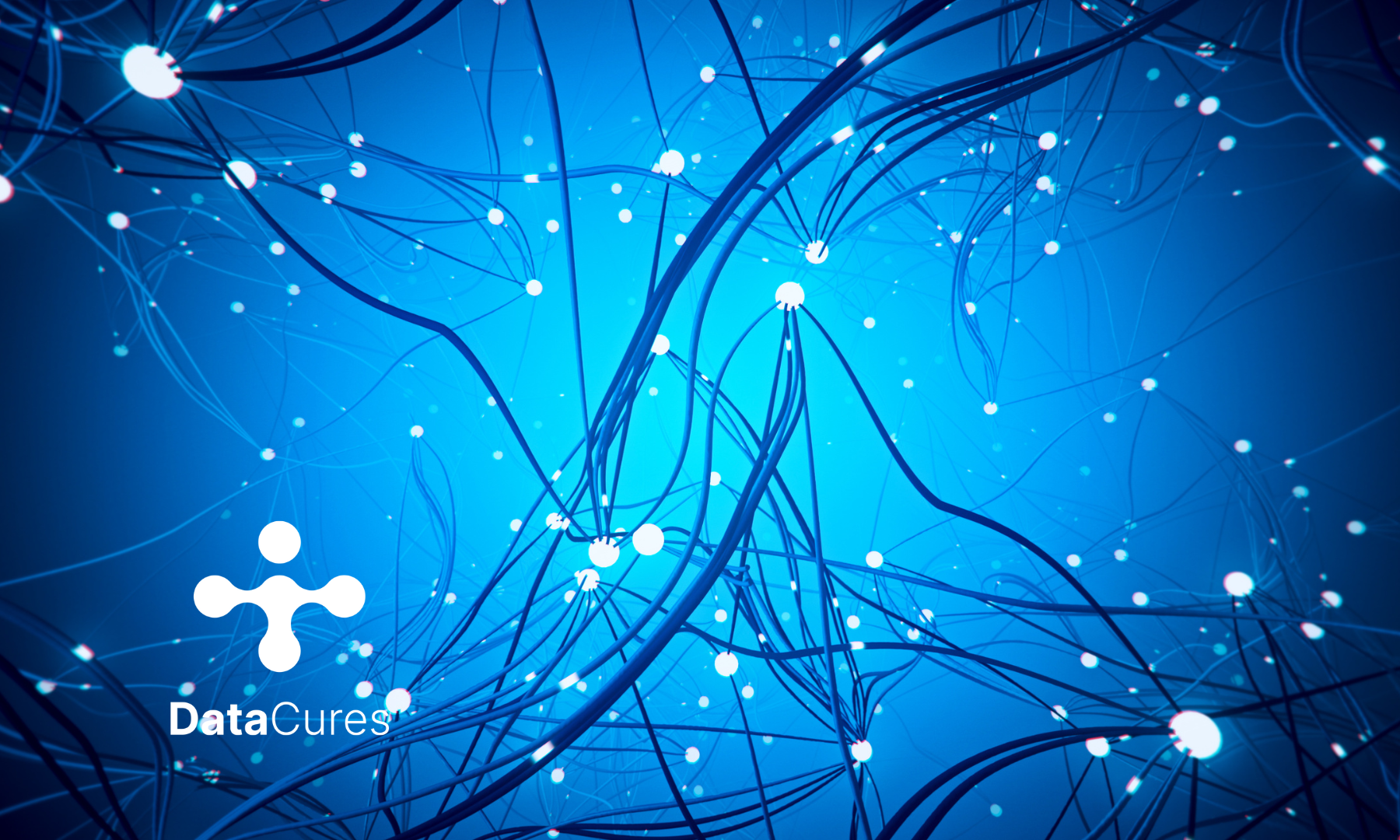
- AI and data science
-
by Nuno Silva
In recent years, the healthcare industry has seen a significant shift towards leveraging emerging technologies to improve patient outcomes. One such technology is the use of sensors and deep learning in remote diagnoses. Sensors are being used to gather data from patients and provide healthcare professionals with real-time insights into their health, while deep learning algorithms are being used to process this data and identify potential health risks. The combination of sensors and deep learning has the potential to revolutionize the way healthcare is delivered, particularly in remote settings where access to medical professionals is limited. This article will explore the exciting possibilities of sensors and deep learning in healthcare and provide real-life examples of how these technologies are already saving lives.
Importance of unlocking the future of healthcare
The use of sensors and deep learning in healthcare has the potential to unlock a future where patients can receive personalized and proactive care rather than reactive care. With the ability to monitor patients remotely and analyze their health data in real-time, healthcare professionals can detect health issues earlier, leading to better outcomes and reduced healthcare costs. Moreover, the use of sensors and deep learning can improve healthcare access for underserved populations, particularly those in remote areas where medical resources are scarce. By leveraging these technologies, healthcare can become more efficient, cost-effective, and patient-centred. As such, unlocking the future of healthcare with sensors and deep learning is crucial for advancing the healthcare industry and improving patient outcomes.
Brief overview of sensors and deep learning in remote diagnoses
Sensors are devices that can measure physical, chemical, or biological parameters and convert them into electrical or digital signals. In healthcare, sensors are being used to monitor various physiological parameters, such as heart rate, blood pressure, and oxygen saturation, among others. These sensors can be integrated into wearable devices, implantable devices, or even smartphones, providing healthcare professionals with real-time data on patient health.
Deep learning, on the other hand, is a subset of machine learning that involves training artificial neural networks to recognize patterns in data. In healthcare, deep learning algorithms are being used to analyze the vast amounts of health data generated by sensors and other sources, such as electronic health records. These algorithms can identify potential health risks, predict outcomes, and even suggest treatment plans.
Together, sensors and deep learning are transforming remote diagnoses by allowing healthcare professionals to monitor patients remotely and detect health issues earlier. This can lead to improved patient outcomes, reduced healthcare costs, and increased access to healthcare for underserved populations.
The Role of Sensors in Healthcare
Definition and explanation of sensors
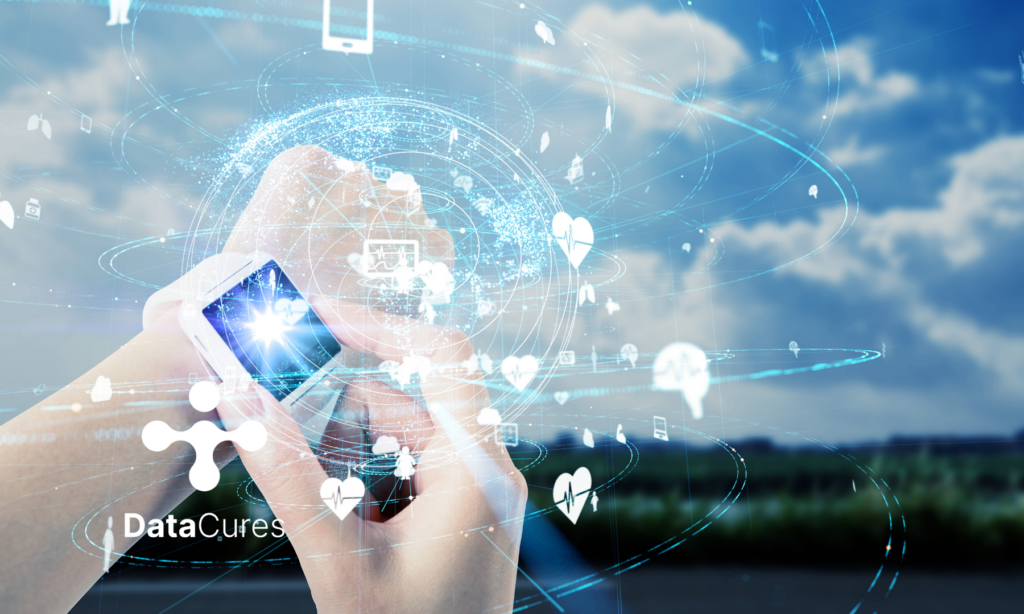
Sensors are devices that can detect and measure physical, chemical, or biological parameters and convert them into electrical or digital signals. In healthcare, sensors are being used to monitor various physiological parameters, such as heart rate, blood pressure, and oxygen saturation, among others. These sensors can be integrated into wearable devices, implantable devices, or even smartphones, providing healthcare professionals with real-time data on patient health.
The use of sensors in healthcare is not new, but recent advances in technology have enabled the development of smaller, more accurate, and more affordable sensors. This has led to a proliferation of wearable devices that can track various health metrics and enable patients to take an active role in monitoring their own health. Sensors are also being used to remotely monitor patients with chronic conditions, such as diabetes or heart disease, enabling healthcare professionals to detect health issues earlier and intervene before they become more serious.
The use of sensors in healthcare has the potential to improve patient outcomes, reduce healthcare costs, and increase access to healthcare, particularly in remote or underserved areas. As such, sensors are playing an increasingly important role in the future of healthcare.
Types of sensors used in healthcare
There are various types of sensors used in healthcare, each with its own specific application. Here are some of the most common types of sensors used in healthcare:
- Electrocardiogram (ECG) sensors: These sensors measure the electrical activity of the heart and are commonly used to diagnose heart conditions such as arrhythmia.
- Blood pressure sensors: These devices can measure blood pressure continuously and provide insights into a patient’s cardiovascular health.
- Oxygen saturation sensors: They measure the amount of oxygen in a patient’s blood and are commonly used to monitor patients with respiratory conditions.
- Glucose sensors: These sensors can measure blood glucose levels continuously, providing patients with diabetes with real-time information on their glucose levels.
- Temperature sensors: They can monitor a patient’s body temperature, which can be useful in detecting fevers or other signs of infection.
- Accelerometers: These devices can detect movement and are commonly used in wearable devices to track activity levels and monitor falls.
- Electroencephalogram (EEG) sensors: These tools are used to measure the electrical activity in the brain and can be used to diagnose neurological conditions such as epilepsy.
The use of these sensors, in combination with deep learning algorithms, is revolutionizing the way healthcare is delivered, enabling healthcare professionals to detect health issues earlier and provide more personalized care to patients.
Real-life examples of sensor technology in healthcare
Sensor technology is being used in healthcare in a variety of ways, from monitoring patient health to enabling remote diagnoses. Here are some real-life examples of sensor technology in healthcare:
- Continuous Glucose Monitoring (CGM): CGM uses a small sensor inserted under the skin to measure glucose levels continuously, providing patients with diabetes with real-time information on their glucose levels.
- Wearable activity trackers: These devices, such as Fitbit or Apple Watch, use accelerometers to track activity levels and provide insights into a patient’s physical activity and sleep patterns.
- Remote monitoring of chronic conditions: Sensors can be used to remotely monitor patients with chronic conditions such as heart disease or COPD, enabling healthcare professionals to detect health issues earlier and intervene before they become more serious.
- Fall detection: Wearable devices equipped with sensors can detect falls and alert caregivers or emergency services, providing patients with greater safety and peace of mind.
- Electronic pill bottles: These devices use sensors to monitor whether patients have taken their medication as prescribed, helping to improve medication adherence and reduce hospital readmissions.
- Smart inhalers: These devices use sensors to monitor inhaler use, providing patients with feedback on their inhaler technique and enabling healthcare professionals to monitor the effectiveness of treatment.
- Remote patient monitoring: Sensors can be used to remotely monitor a patient’s vital signs, such as heart rate, blood pressure, and respiratory rate, allowing healthcare professionals to monitor patients who are recovering at home after surgery or who have chronic conditions.
- Digital stethoscopes: These devices use sensors to amplify and filter sounds from the heart, lungs, and other organs, allowing healthcare professionals to better diagnose and treat respiratory and cardiovascular conditions.
- Smart beds: These beds use sensors to monitor a patient’s position, movement, and weight distribution, providing insights into patient mobility and pressure ulcers.
- Wireless blood pressure monitors: These devices use sensors to measure blood pressure and can send the data to a smartphone app or cloud platform, allowing patients to monitor their blood pressure at home and share the data with their healthcare provider.
The use of sensor technology in healthcare is still in its early stages, but the potential benefits are significant, including improved patient outcomes, reduced healthcare costs, and increased access to healthcare for underserved populations.
Advantages and challenges of using sensors in healthcare
The use of sensors in healthcare has many potential advantages, including:
- Improved patient outcomes: Sensors can provide healthcare professionals with real-time data on patient health, enabling earlier detection and treatment of health issues.
- Increased access to healthcare: Remote monitoring using sensors can enable patients to receive care from anywhere, reducing the need for in-person visits and increasing access to healthcare for underserved populations.
- Reduced healthcare costs: By enabling earlier detection and treatment of health issues, sensors can help to reduce the cost of healthcare by preventing hospitalizations and reducing the need for expensive treatments.
- Better patient engagement: Sensors can provide patients with real-time feedback on their health status, enabling them to take a more active role in managing their health.
However, there are also several challenges associated with using sensors in healthcare, including:
- Privacy and security concerns: Using sensors generates large amounts of personal health data, which must be stored securely and protected from unauthorized access.
- Technical challenges: Sensors must be designed and calibrated correctly to ensure accurate data collection and interpretation.
- Integration with existing systems: Integrating sensor technology with existing healthcare systems and processes can be challenging, requiring changes to workflows and the adoption of new technologies.
- Cost: The cost of sensor technology can be a barrier to adoption, particularly for smaller healthcare providers or patients with limited resources.
Despite these challenges, the potential benefits of using sensors in healthcare are significant, and the technology is likely to play an increasingly important role in the future of healthcare.
Deep Learning in Healthcare
Definition and explanation of deep learning
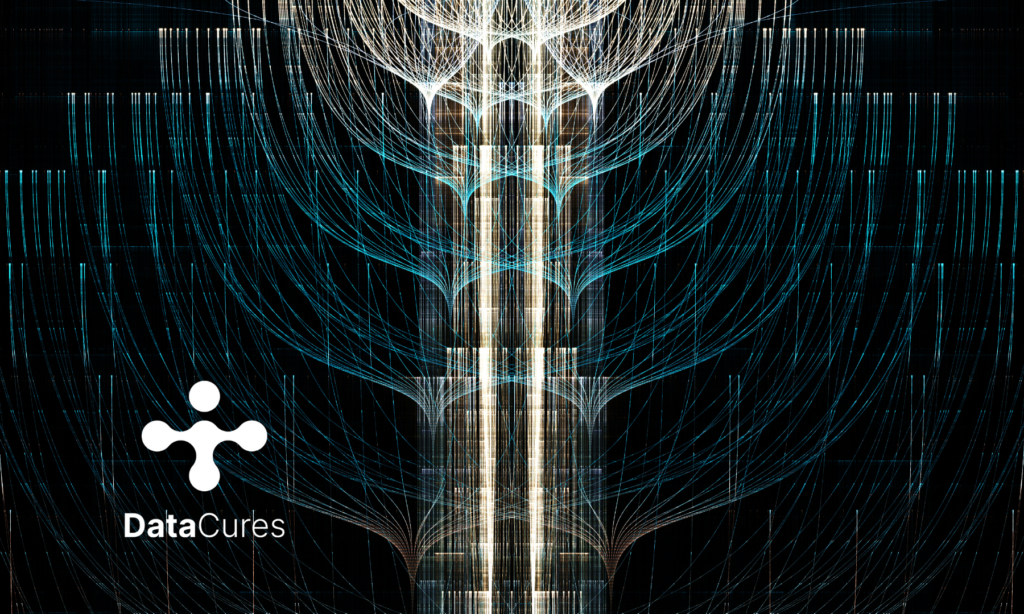
Deep learning is a subfield of machine learning that uses neural networks to model and solve complex problems. It is based on the idea of creating artificial neural networks that simulate the functioning of the human brain. These networks are composed of layers of interconnected nodes, each of which processes and transmits information to the next layer.
Deep learning algorithms use these neural networks to learn from large datasets, identifying patterns and relationships in the data that can be used to make predictions or classify new data. The ability of deep learning algorithms to automatically identify and learn from patterns in data has made them particularly useful in healthcare, where large amounts of data are generated every day, and the ability to analyze this data quickly and accurately can have a significant impact on patient outcomes.
Overall, deep learning has the potential to revolutionize healthcare by enabling faster and more accurate diagnosis, improving patient outcomes, and reducing healthcare costs.
Real-life examples of deep learning in healthcare
Deep learning has already been successfully applied in a variety of healthcare settings, with promising results. Here are some real-life examples of deep learning in healthcare:
- Radiology: Deep learning algorithms have been applied to radiology to help identify and classify medical images, such as X-rays and CT scans. For example, a team of researchers at Stanford University developed a deep learning algorithm that can detect pneumonia in chest X-rays with a high degree of accuracy.
- Early detection of diabetic retinopathy: Google developed a deep learning algorithm that can detect diabetic retinopathy, a complication of diabetes that can lead to blindness if left untreated, with a high degree of accuracy. The algorithm was tested in India, where it was used to screen patients for diabetic retinopathy, enabling earlier detection and treatment of the condition.
- Early detection of Alzheimer’s disease: Researchers at the University of California, San Francisco, developed a deep learning algorithm that can predict the onset of Alzheimer’s disease up to six years in advance, using data from brain scans. This technology has the potential to enable earlier detection and treatment of the disease, improving patient outcomes.
These examples demonstrate the potential of deep learning to improve healthcare outcomes by enabling faster and more accurate diagnosis, and by providing new insights into complex medical conditions. However, the adoption of these technologies must be accompanied by appropriate safeguards to ensure patient privacy and data security.
Advantages and challenges of using deep learning in healthcare
Deep learning has the potential to revolutionize healthcare by enabling faster and more accurate diagnosis, as well as providing new insights into complex medical conditions. Here are some advantages and challenges of using deep learning in healthcare:
Advantages:
- Faster and more accurate diagnosis: Deep learning algorithms can analyze vast amounts of medical data, such as medical images and patient records, much faster than humans can and with greater accuracy. This can lead to faster and more accurate diagnoses, as well as more personalized treatment plans.
- Improved patient outcomes: By enabling earlier and more accurate diagnoses, deep learning has the potential to improve patient outcomes and reduce healthcare costs by enabling more efficient use of healthcare resources.
- Enhanced research capabilities: Deep learning can help researchers identify patterns and relationships in complex medical data that would be difficult or impossible for humans to detect. This can lead to new insights into medical conditions and more effective treatments.
Challenges:
- Data privacy and security: Deep learning algorithms require large amounts of sensitive medical data to train and improve their accuracy. This raises concerns about patient privacy and data security, particularly in the context of data breaches and cyber-attacks.
- Lack of interpretability: Deep learning algorithms are often described as “black boxes” because it can be difficult to understand how they arrive at their decisions. This can make it challenging to identify errors or biases in the algorithm and to communicate the reasoning behind medical decisions to patients.
- Integration with existing systems: Deep learning algorithms may require significant changes to existing healthcare systems and workflows, which can be costly and time-consuming.
Despite these challenges, the potential benefits of deep learning in healthcare are significant, and continued research and development in this area are likely to lead to new breakthroughs in medical diagnosis and treatment.
Ethical and Legal Considerations
Explanation of ethical and legal considerations related to sensor and deep learning technologies
As with any new technology, the use of sensors and deep learning in healthcare raises important ethical and legal considerations that must be carefully considered. Here are some of the key issues to consider:
- Privacy and data security: The use of sensors and deep learning in healthcare requires the collection and storage of large amounts of sensitive patient data. This raises concerns about patient privacy and data security, particularly in the context of data breaches and cyber-attacks.
- Informed consent: Patients must be fully informed about the use of sensors and deep learning in their healthcare and must be given the opportunity to consent or refuse to participate. This raises questions about how to provide patients with clear and accurate information about complex technologies and how to ensure that consent is truly informed.
- Bias and fairness: Deep learning algorithms can be susceptible to bias, particularly if they are trained on data that is not representative of the population as a whole. This raises concerns about fairness and the potential for discrimination in healthcare.
- Liability and accountability: The use of sensors and deep learning in healthcare raises questions about liability and accountability in the event of errors or malfunctions. Who is responsible when a sensor or algorithm makes a mistake that results in harm to a patient?
- Regulation and oversight: The use of sensors and deep learning in healthcare raises questions about regulation and oversight. How can we ensure that these technologies are developed and used in ways that are safe, effective, and ethical?
By addressing these ethical and legal considerations, the use of sensors and deep learning in healthcare can be guided by principles of transparency, fairness, and respect for patient privacy and autonomy.
Conclusion
Summary of the article
In this article, we have explored the role of sensors and deep learning in healthcare and their potential to revolutionize the way we diagnose and treat medical conditions. We have provided a brief overview of sensors and deep learning technologies, discussed real-life examples of their applications in healthcare, and examined their advantages and challenges.
We have also highlighted the ethical and legal considerations that arise with the use of these technologies, including privacy, informed consent, bias and fairness, liability, and regulation. By addressing these considerations, we can ensure that the use of sensors and deep learning in healthcare is guided by principles of transparency, fairness, and respect for patient privacy and autonomy.
In conclusion, the use of sensors and deep learning in healthcare offers exciting possibilities for improving patient outcomes and reducing healthcare costs. By continuing to innovate and address the ethical and legal considerations that arise with their use, we can unlock the full potential of these technologies and improve the future of healthcare.
Exciting possibilities for the future of healthcare
The use of sensors and deep learning in healthcare has the potential to bring about significant advancements in medical diagnosis, treatment, and care. Some of the most exciting possibilities include:
- Personalized medicine: With the use of sensors and deep learning algorithms, doctors can create personalized treatment plans based on individual patient data, including genetic and environmental factors.
- Early disease detection: Sensors can monitor patients in real time, enabling early detection of diseases and conditions before they become severe. This can lead to earlier treatment and better outcomes for patients.
- Remote patient monitoring: With the help of sensors and deep learning, healthcare professionals can remotely monitor patients in their homes, reducing the need for hospital visits and improving patient comfort and convenience.
- Predictive analytics: Deep learning algorithms can analyze vast amounts of patient data, allowing healthcare providers to make accurate predictions about patient health and identify potential health risks.
- Improved medical imaging: Deep learning algorithms can improve the accuracy of medical imaging, helping doctors to identify and diagnose medical conditions more accurately.
These possibilities represent just a few of the many ways in which sensors and deep learning can improve the future of healthcare. As technology continues to evolve and improve, we can expect to see even more exciting developments in this field.
About the author:
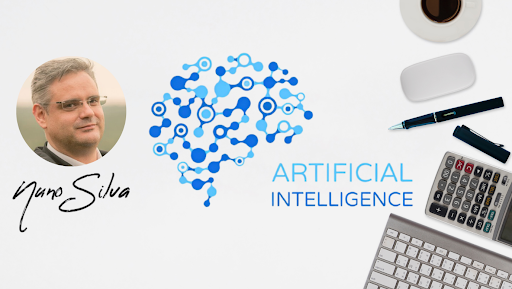
Nuno Silva is the Chief Scientific and Technology Officer at UnifAI Technology. He is a recognized thought leader in the AI and healthcare industries, with multiple publications and speaking engagements to his credit. He is passionate about using technology to improve quality of life.